c:o/re Senior Fellow 09/23 − 06/24
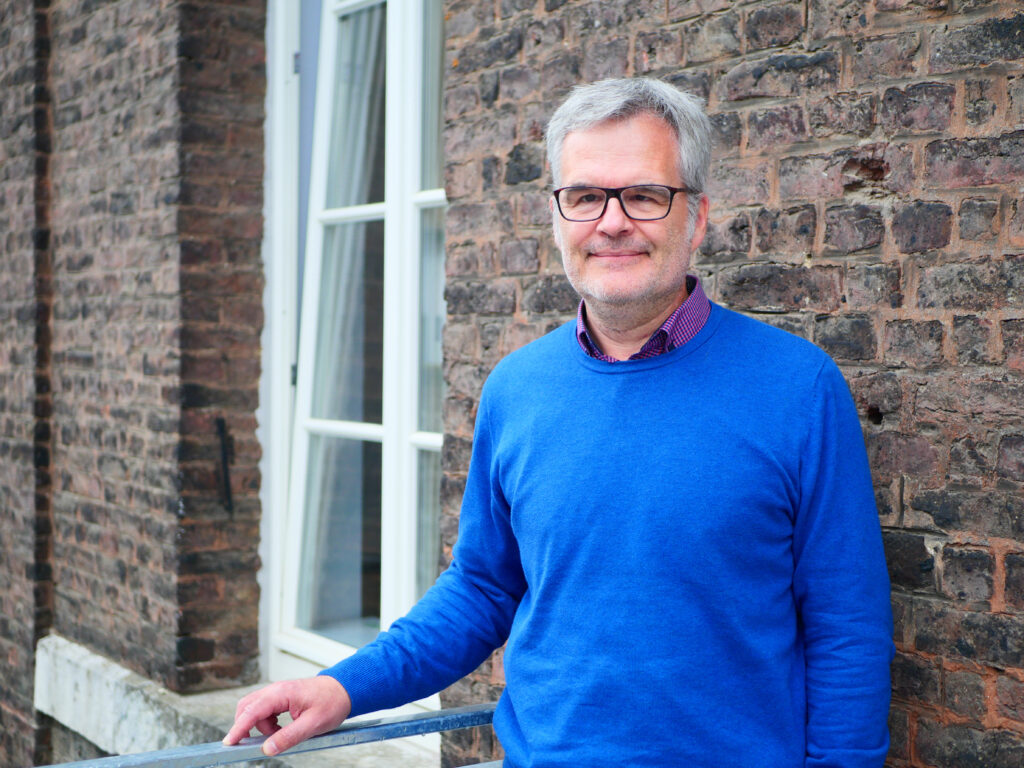
Hans Ekkehard Plesser received his diploma in physics from RWTH Aachen in 1995. He started working on computational neuroscience at RIKEN (Japan) the following year, and obtained his doctoral degree from Göttingen University in 1999. Since 2000, Hans Ekkehard has been at the Norwegian University of Life Sciences (NMBU) first as a Marie-Curie Fellow, then as associate professor and since 2018 as full professor in informatics. He has served multiple terms as department head at the Faculty of Science and Technology at NMBU. Hans Ekkehard focuses on simulation technology for large-scale neuronal network simulations and reproducibility of research in computational neuroscience.
He has been a visiting researcher at New York University, Ohio State University, RIKEN Brain Science Institute, Simula Research Laboratory and University of Oslo, and is now a guest scientist at Forschungszentrum Jülich. From 2013 to 2020, he served as chairman of the board of the Norwegian Research School for Neuroscience.
For more than 20 years, Hans Ekkehard has been a lead developer of the NEST Simulator, being a founding member and president of the NEST Initiative. Through a wide range of roles in the European Flagship Human Brain Project since 2013, he has acquired considerable insight not only into the content but also the process of neuroscience.
Model divergence: On the lack of synthesis in computational neuroscience
Convergence is a hallmark of models in physics: Newton’s laws of mechanics explained planetary motion as well as falling fruit, while Bohr’s model of the atom provided a concise explanation of a wide range of spectral lines and stimulated the development of quantum mechanics. The failure to reconcile relativity theory and quantum mechanics, which would be required for combining all fundamental forces in a single standard model, is considered a key problem of physics today.
Explaining the brain is an altogether different task than explaining planetary motion. Classical physics owes much of its success to the separation of scales, which allows physicists to study laws of motion for idealized point masses with no or very few internal degrees of freedom. The nervous system, in contrast, consists of tightly connected complex entities, suggesting no obvious level of abstraction for studying brain function.
Computational neuroscience displays a very different research culture to that of physics. Computational neuroscientists appear to prefer to develop a steadily growing collection of models of neurons (nerve cells), of synapses (connections between neurons) and of networks of neurons, instead of systematically integrating existing models.
In my project, I will chart the trend of model divergence in computational neuroscience, attempt to identify its underlying causes, and develop examples of how to overcome it. A key question I will address in this context is whether model divergence may have resulted from a lack of digital tools suited to capture the complexity of brain models.
The first goal of my study is to analyze to which degree published models of neurons, synapses and networks have been picked up and re-used by scientists other than their original authors. The second goal is to evaluate if some exemplary models could be described conceptually in a concise way that allows for their re-use, e.g., with reference to ontologies, more than merely being shared in form of source code. The third goal of my project consist in an exemplary comparison of network models to identify which properties of neuron and synapse models are essential to obtain relevant dynamical properties of networks.
Publications (Selection)
J. Senk, B. Kriener, M. Djurfeldt, N. Voges, H.-J. Jiang, L. Schüttler, G. Gramelsberger, M. Diesmann, H. E. Plesser, and S. J. v Albada. 2022. Connectivity concepts in neuronal network modeling. In PLOS Comput Biol, 18:1-49. DOI 10.1371/journal.pcbi.1010086.
G. T. Einevoll, A. Destexhe, M. Diesmann, S. Grün, V. Jirsa, M. d Kamps, M. Migliore, T. V. Ness, H. E. Plesser, and F. Schürmann. 2019. The scientific case for brain simulations. In
Neuron, 102(4):735-744. DOI 10.1016/j.neuron.2019.03.027. On arXiv.org.
Hans Ekkehard Plesser. 2018. Reproducibility vs. replicability: A brief history of a confused terminology. In Frontiers in Neuroinformatics, 11:76. DOI 10.3389/fninf.2017.00076.
S. Crook, J. Bednar, S. Berger, R. Cannon, A. Davison, M. Djurfeldt, J. Eppler, B. Kriener, S. Furber, B. Graham, H. E. Plesser, L. Schwabe, L. Smith, V. Steuber, and S. v Albada. 2012. Creating, documenting and sharing network models. In Network-Comp Neural, 23:131-149. Preprint.
E. Nordlie, M.-O. Gewaltig, and H. E. Plesser. 2009. Towards reproducible descriptions of neuronal network models. In PLoS Comput Biol, 5(8):e1000456. DOI 10.1371/journal.pcbi.1000456.